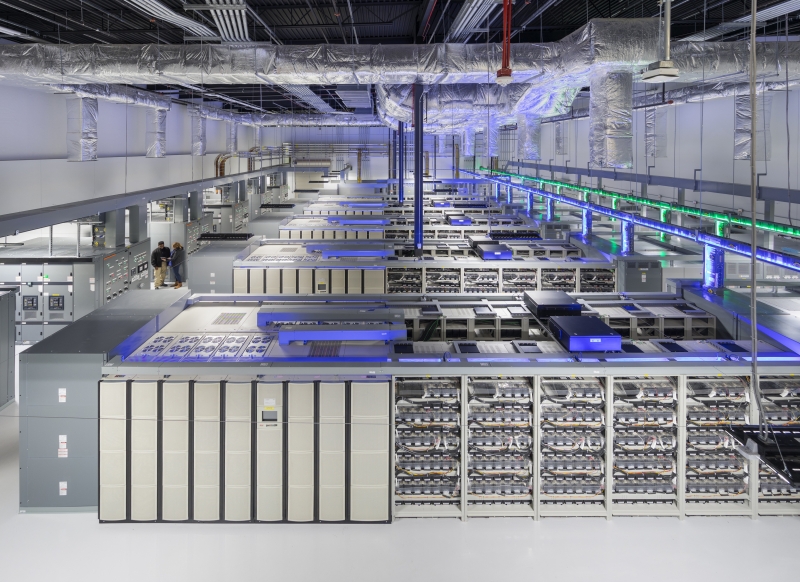
Data centers stand as the modern equivalent of libraries, silently housing vast stores of information that power our interconnected world. Here, rows upon rows of towering, metallic racks blink with LEDs, a digital dance that reveals the heartbeat of the devices. Each row of lights represents a server – a high-powered computer dedicated to processing the intricate tapestry of ones and zeros that make up the language of digital data transmission. These data centers, with their vast amounts of stored information and unfathomable computing power, play a pivotal role in advancing Artificial Intelligence (AI) and the digital age.
As AI is reshaping how we interact with machines and the world around us, the design of data centers needs to adapt to this fast-changing landscape. So, Page pairs expert thinking with high-performing solutions to meet the needs of rapidly advancing technologies.
How does AI learn?
In simple terms, AI learns from data, often adapting and improving its performance over time. It then applies this knowledge to situations the model hasn’t seen before. We call these two tasks – the process of learning and applying knowledge – training (or machine learning) and inference.
During training, AI models are exposed to a massive amount of data, discovering patterns and correlations. Just as a child learns to recognize shapes and colors by exposure, AI learns to understand complex data. Training requires extensive datasets, so data centers provide the necessary infrastructure to store, manage, and deliver the information to the models. In the inference stage, AI applies the knowledge gained from the training stage to make decisions about new data.
Futureproofing Data Center Design
For the past decade, data centers have been tailored to meet the needs of the cloud computing era. The facilities are massive warehouses filled with powerful computers that operate independently from one another. Training at scale requires these larger, centralized data centers as well. However, the inference phase is moving towards edge computing – a decentralized approach to data processing that brings decision-making closer to the data source, reducing latency while enhancing real-time processing.
Now, stakeholders, architects, and engineers must reimagine the traditional design of data centers to accommodate the needs of each phase better. And there is no one-size-fits-all approach for data centers. So, Page creates customized, AI-optimized ecosystems that are dynamic, responsive, and ever-evolving.
The rapid innovation in AI hardware designs requires a dynamic, adaptable facility. Imagine a digital ecosystem that responds like a living organism, growing and evolving in tandem with the demands placed on it; this is the essence of modular design.
Modular design – when a facility is comprised of smaller, self-contained components – provides an opportunity for a seamless expansion that enhances a building’s performance and capacity. For example, rows of servers can be flexibly connected to accommodate an increasing number of AI workloads, like an ever-expanding library. This approach allows data centers grow organically, adding computing nodes, storage arrays, and networking equipment like Lego pieces snapping into place. This scalable growth defines the success of data centers in today’s environment.
Page used a step-by-step expansion plan in an Iowa data center that didn’t require significant structural modifications. With phased delivery of each data hall, we incrementally increased the critical IT capacity growth to incorporate new technology. Using prefabricated components, the electrical design also accommodates a scalable build-out that is more adaptable for future changes or expansions.
Rethinking Cooling Solutions
These data-hungry machines also have a large appetite for energy. And traditional HVAC solutions are less effective for cooling hardware with increased heat densities. AI models are trained on specialized hardware that draws more power than traditional servers, and this surge in consumption inevitably leads to increased heat production.
Immersion and liquid cooling, which use fluid to absorb and dissipate heat, are more streamlined, direct, and efficient cooling methods. Liquid is a more efficient heat conductor than air, so it can quickly absorb and transport heat away from sensitive components. Welcoming machine learning, existing data centers must strategically transition from air-cooled servers to immersion or liquid cooling solutions without sacrificing the high efficiency that data center users and operators depend on.
The possibilities for data center design are boundless, with increasingly efficient technologies driving innovation. And Page understands, in the ever-evolving digital world, the need for adaptable, scalable, and efficient design has never been more crucial.